Outlier Scale AI is revolutionizing the way we analyze data, offering a robust framework for identifying anomalies and enhancing decision-making processes. As the digital landscape continues to expand, organizations are increasingly relying on advanced AI techniques to glean insights from vast amounts of data. Outlier detection becomes critical in this context, enabling businesses to spot irregularities that could indicate fraud, operational inefficiencies, or emerging trends.
In this article, we will explore the concept of Outlier Scale AI, its significance in data analytics, and how it is applied across various industries. We will delve into the methodologies behind outlier detection, the benefits it offers, and the future of this technology. By the end of this discussion, you will have a comprehensive understanding of how Outlier Scale AI can be leveraged to enhance your data analysis capabilities.
Whether you are a data scientist, a business analyst, or a decision-maker, understanding Outlier Scale AI will equip you with the knowledge needed to utilize this powerful tool effectively. Let’s embark on this journey to uncover the potentials of this transformative technology.
Table of Contents
- What is Outlier Scale AI?
- Importance of Outlier Detection
- Methodologies for Outlier Detection
- Applications in Different Industries
- Benefits of Outlier Scale AI
- Challenges and Limitations
- Future of Outlier Scale AI
- Conclusion
What is Outlier Scale AI?
Outlier Scale AI refers to the application of artificial intelligence techniques specifically designed to identify and analyze outliers within large datasets. Outliers are data points that deviate significantly from the mean or expected patterns, and detecting them is crucial for various analytical tasks. Outlier Scale AI employs algorithms that leverage machine learning and statistical methods to automate the detection process, making it faster and more efficient.
Key Characteristics of Outlier Scale AI
- Scalability: Capable of processing and analyzing large datasets effectively.
- Automation: Reduces the need for manual intervention in identifying outliers.
- Real-time Analysis: Provides immediate insights into data anomalies.
- Integration: Can be integrated with existing data analysis tools and frameworks.
Importance of Outlier Detection
Detecting outliers is vital for several reasons. It helps organizations to maintain data integrity and ensures that decisions are based on accurate information. Here are some of the key reasons why outlier detection is essential:
- Fraud Detection: Identifying unusual transactions that may indicate fraudulent activity.
- Quality Control: Spotting defects or errors in manufacturing processes.
- Trend Analysis: Recognizing emerging trends that may deviate from historical data.
- Risk Management: Assessing potential risks by analyzing data anomalies.
Methodologies for Outlier Detection
Outlier detection can be achieved through various methodologies, each suited to different types of data and use cases. Here are some of the most common approaches:
Statistical Methods
Statistical methods involve using statistical tests to identify outliers. Techniques such as Z-score and the Tukey method are commonly employed. These methods work well for normally distributed data.
Machine Learning Techniques
Machine learning techniques, including supervised and unsupervised learning, are increasingly used for outlier detection. Algorithms like Isolation Forest, One-Class SVM, and Autoencoders can effectively identify anomalies in complex datasets.
Applications in Different Industries
Outlier Scale AI has a wide range of applications across various industries. Here are a few notable examples:
- Finance: Monitoring transactions for signs of fraud and assessing credit risk.
- Healthcare: Identifying unusual patient data that may indicate errors in diagnosis or treatment.
- Retail: Analyzing consumer behavior to detect anomalies in purchasing patterns.
- Manufacturing: Monitoring production lines for defects and inefficiencies.
Benefits of Outlier Scale AI
The implementation of Outlier Scale AI offers numerous benefits for organizations, including:
- Enhanced Decision Making: Provides deeper insights that lead to more informed decisions.
- Increased Efficiency: Automates the detection process, saving time and resources.
- Improved Data Quality: Helps maintain data integrity by identifying erroneous data points.
- Competitive Advantage: Enables organizations to act quickly on emerging trends and anomalies.
Challenges and Limitations
Despite its advantages, Outlier Scale AI also faces several challenges and limitations:
- Data Quality: Poor quality data can lead to inaccurate outlier detection.
- Complexity: Implementing sophisticated algorithms requires expertise and resources.
- Interpretability: Some machine learning models may be difficult to interpret, making it hard to understand the reasoning behind detected outliers.
- Dynamic Data: Data patterns may change over time, requiring continuous model updates.
Future of Outlier Scale AI
The future of Outlier Scale AI looks promising as advancements in artificial intelligence and machine learning continue to evolve. We can expect:
- Integration with Big Data Technologies: Enhanced capabilities for processing and analyzing massive datasets.
- Development of More Robust Algorithms: Improved accuracy and efficiency in outlier detection.
- Greater Accessibility: Tools and platforms becoming more user-friendly for non-experts.
- Real-Time Analytics: Increased focus on real-time outlier detection for immediate action.
Conclusion
Outlier Scale AI is a powerful tool that transforms how organizations analyze and interpret data. By effectively detecting anomalies, businesses can enhance decision-making, improve operational efficiency, and maintain data integrity. As technology continues to advance, the capabilities and applications of Outlier Scale AI will likely expand, offering even greater benefits. We encourage you to explore how Outlier Scale AI can be integrated into your data analysis processes and share your thoughts in the comments below. Don't forget to share this article with others who may find it useful!
Final Thoughts
Thank you for reading this article on Outlier Scale AI. We hope you found it informative and engaging. Stay tuned for more insights and discussions on the latest trends in data analytics and artificial intelligence. We look forward to welcoming you back to our site for more valuable content.
Understanding Trump Adin: A Comprehensive Guide
Wuthering Waves Radiant Tide: Exploring The Depths Of Nature's Beauty
Exploring "うい麦畑でつかまえて" By しぐれうい: A Journey Through Music And Emotion


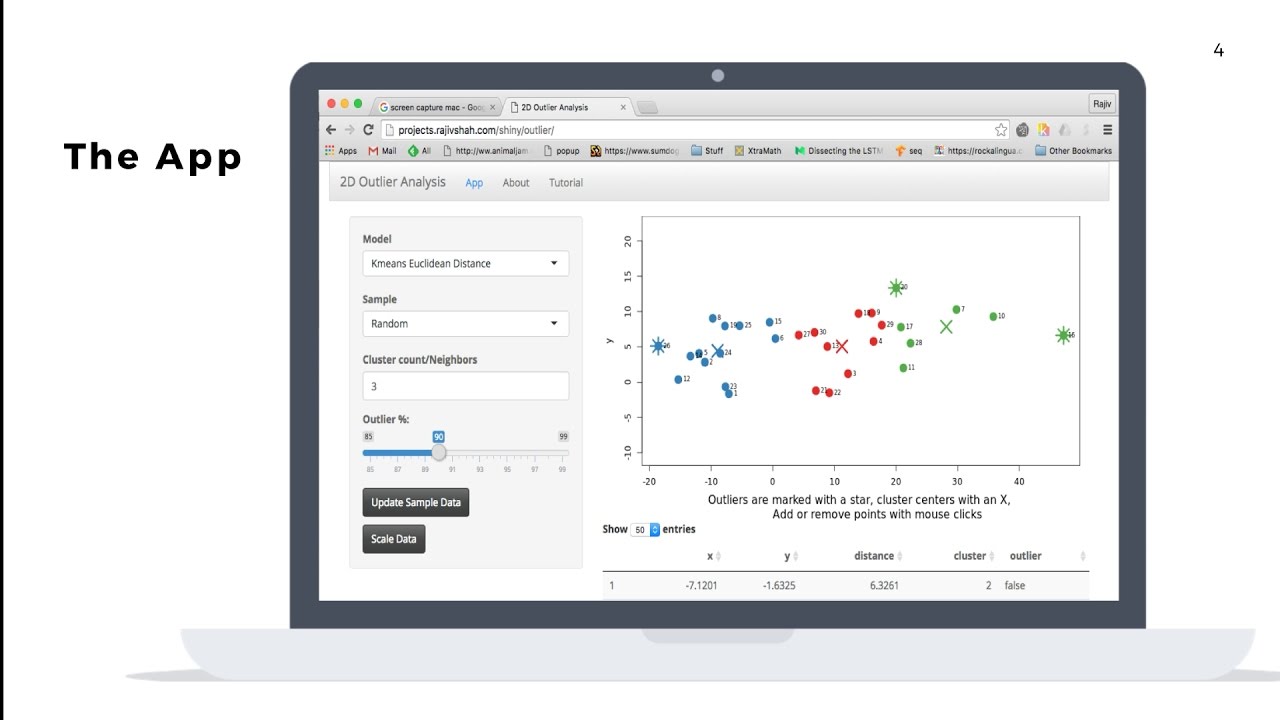